Carry this challenge to life
Within the Gen-AI world, now you can experiment with totally different hairstyles and create a artistic search for your self. Whether or not considering a drastic change or just looking for a recent look, the method of imagining oneself with a brand new coiffure could be each thrilling and daunting. Nevertheless, with using synthetic intelligence (AI) know-how, the panorama of hairstyling transformations is present process a groundbreaking revolution.
Think about having the ability to discover an countless array of hairstyles, from basic cuts to 90’s designs, all from the consolation of your individual house. This futuristic fantasy is now a potential actuality due to AI-powered digital hairstyling platforms. By using the facility of superior algorithms and machine studying, these progressive platforms enable customers to digitally attempt on numerous hairstyles in real-time, offering a seamless and immersive expertise not like something seen earlier than.
On this article, we’ll discover HairFastGAN and perceive how AI is revolutionizing how we experiment with our hair. Whether or not you are a magnificence fanatic desirous to discover new developments or somebody considering a daring hair makeover, be a part of us on a journey via the thrilling world of AI-powered digital hairstyles.
Introduction
This paper introduces HairFast, a novel mannequin designed to simplify the complicated activity of transferring hairstyles from reference pictures to private photographs for digital try-on. In contrast to present strategies which can be both too sluggish or sacrifice high quality, HairFast excels in velocity and reconstruction accuracy. By working in StyleGAN’s FS latent house and incorporating enhanced encoders and inpainting methods, HairFast efficiently achieves high-resolution leads to close to real-time, even when confronted with difficult pose variations between supply and goal pictures. This strategy outperforms present strategies, delivering spectacular realism and high quality, even when transferring coiffure form and colour in lower than a second.
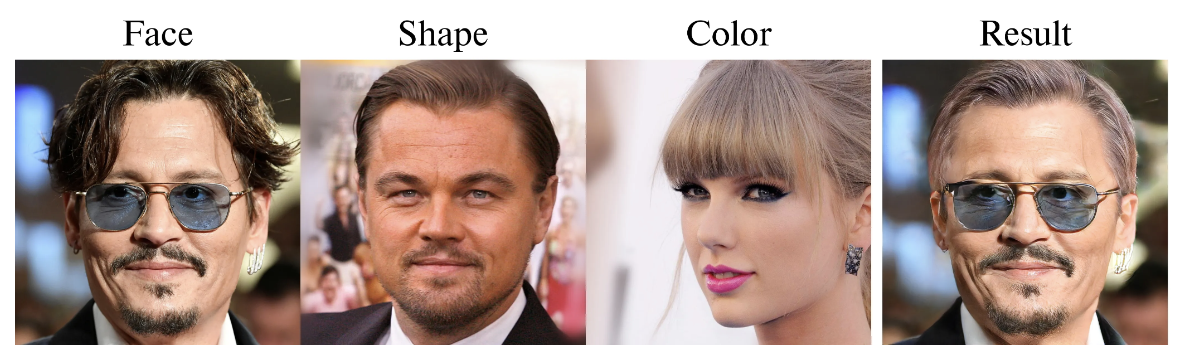
Because of developments in Generative Adversarial Networks (GANs), we are able to now use them for semantic face modifying, which incorporates altering hairstyles. Coiffure switch is a very difficult and interesting facet of this subject. Basically, it includes taking traits like hair colour, form, and texture from one picture and making use of them to a different whereas protecting the individual’s identification and background intact. Understanding how these attributes work collectively is essential for getting good outcomes. This type of modifying has many sensible makes use of, whether or not you are an expert working with picture modifying software program or simply somebody enjoying digital actuality or laptop video games.
The HairFast methodology is a quick and high-quality resolution for altering hairstyles in photographs. It might probably deal with high-resolution pictures and produces outcomes similar to the most effective present strategies. It is also fast sufficient for interactive use, due to its environment friendly use of encoders. This methodology works in 4 steps: embedding, alignment, mixing, and post-processing. Every step is dealt with by a particular encoder skilled to do this particular job.
Latest developments in Generative Adversarial Networks (GANs), like ProgressiveGAN, StyleGAN, and StyleGAN2, have vastly improved picture era, significantly in creating extremely real looking human faces. Nevertheless, attaining high-quality, totally managed hair modifying stays a problem as a consequence of numerous complexities.
Completely different strategies handle this problem in numerous methods. Some deal with balancing editability and reconstruction constancy via latent house embedding methods, whereas others, like Barbershop, decompose the hair switch activity into embedding, alignment, and mixing subtasks.
Approaches like StyleYourHair and StyleGANSalon goals for better realism by incorporating native type matching and pose alignment losses. In the meantime, HairNet and HairCLIPv2 deal with complicated poses and various enter codecs.
Encoder-based strategies, similar to MichiGAN and HairFIT, velocity up runtime by coaching neural networks as an alternative of utilizing optimization processes. CtrlHair, a standout mannequin, makes use of encoders to switch colour and texture, however nonetheless faces challenges with complicated facial poses, resulting in sluggish efficiency as a consequence of inefficient postprocessing.
General, whereas important progress has been made in hair modifying utilizing GANs, there are nonetheless hurdles to beat for attaining seamless and environment friendly leads to numerous eventualities.
Methodology Overview
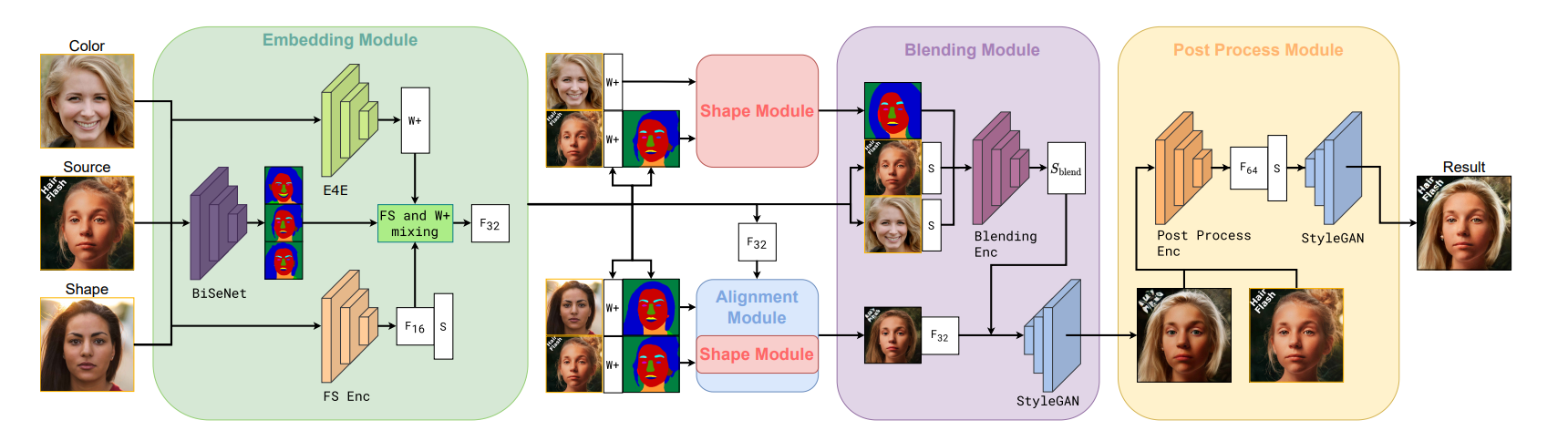
This novel methodology for transferring hairstyles is similar to the Barbershop strategy nevertheless—all optimization processes are changed with skilled encoders for higher effectivity. Within the Embedding module, unique pictures illustration are captured in StyleGAN areas, like W+ for modifying and F S house for detailed reconstruction. Moreover, face segmentation masks are used for later use.
Transferring to the Alignment module, the form of the coiffure from one picture to a different is especially completed by specializing in altering the tensor F. Right here, two duties are accomplished: producing the specified coiffure form through the Form Module and adjusting the F tensor for inpainting post-shape change.
Within the Mixing module, the shift of hair colour from one picture to a different is completed . By modifying the S house of the supply picture utilizing the skilled encoder, that is achieved whereas contemplating further embeddings from the supply pictures.
Though the picture post-blending may very well be thought-about closing, a brand new Submit-Processing module is required. This step goals to revive any misplaced particulars from the unique picture, guaranteeing facial identification preservation and methodology realism enhancement.
Embedding
To begin altering a coiffure, first pictures are transformed into StyleGAN house. Strategies like Barbershop and StyleYourHair do that by reconstructing every picture in F S house via an optimization course of. As a substitute, on this analysis a pre-trained FS encoder is used that shortly provides the F S representations of pictures. It is the most effective encoders on the market and makes pictures look actually good.
However this is the problem: F S house is not straightforward to work with. When altering hair colour utilizing the FS encoder in Barbershop, it does not do an amazing job. So, one other encoder referred to as E4E is used. It is easy and never nearly as good at making pictures look good, nevertheless it’s nice for making modifications. Subsequent, the F tensor (which holds the details about the hair) from each encoders is combined to unravel this drawback.
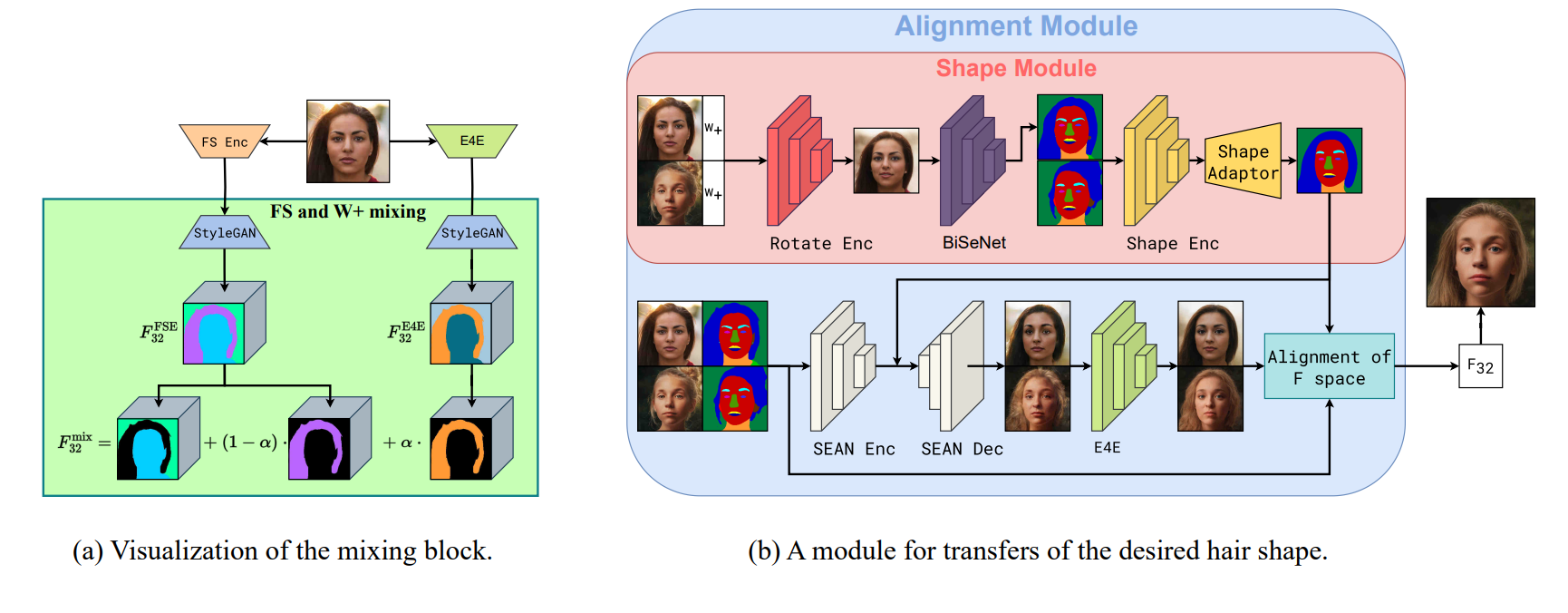
Alignment
On this step, the hair makeover is completed, so the hair in a single image ought to appear like the hair in one other image. To do that, a masks is created that outlines the hair, after which the hair within the first image is adjusted to match that masks.
Some sensible people got here up with a method to do that referred to as CtrlHair. They use a Form Encoder to grasp the shapes of hair and faces in footage and a Form Adaptor to regulate the hair in a single image to match the form of one other. This methodology normally works fairly properly, nevertheless it has some points.
One massive drawback is that the Form Adaptor is skilled to deal with hair and faces in related poses. So if the poses are actually totally different between the 2 footage, it will possibly mess issues up, making the hair look bizarre. The CtrlHair workforce tried to repair this by tweaking the masks afterwards, nevertheless it’s not essentially the most environment friendly resolution.
To sort out this challenge, an extra device referred to as Rotate Encoder was developed. It is skilled to regulate the form picture to match the pose of the supply picture. That is primarily completed by tweaking the illustration of the picture earlier than segmenting it. There is no such thing as a must fine-tune the small print for creating the masks, so a simplified illustration is used on this case. This encoder is skilled to deal with complicated poses with out distorting the hair. If the poses already match, it will not mess up the hairstyles.
Mixing
Within the subsequent step, the primary focus is on altering the hair colour to the specified shade. Beforehand, as we all know Barbershop’s earlier methodology that was too inflexible, looking for a stability between the supply and desired colour vectors. This usually resulted in incomplete edits and added undesirable artifacts as a consequence of outdated optimization methods.
To enhance this, the same encoder structure referred to as HairCLIP is added predicts how the type of the hair vector modifications when given two enter vectors. This methodology makes use of particular modulation layers which can be extra steady and nice for altering kinds.
Moreover, we’re feeding our mannequin with CLIP embeddings of each the supply picture (together with hair) and the hair-only a part of the colour picture. This further data helps protect particulars which may get misplaced through the embedding course of and has been proven to considerably improve the ultimate consequence, based on our experiments.
Experiments Outcomes
The experiments revealed that whereas the CtrlHair methodology scored the most effective based on the FID metric, it truly did not carry out as properly visually in comparison with different state-of-the-art approaches. This discrepancy happens as a consequence of its post-processing method, which concerned mixing the unique picture with the ultimate consequence utilizing Poisson mixing. Whereas this strategy was favored by the FID metric, it usually resulted in noticeable mixing artifacts. Then again, the HairFast methodology had a greater mixing step however struggled with instances the place there have been important modifications in facial hues. This made it difficult to make use of Poisson mixing successfully, because it tended to emphasise variations in shades, resulting in decrease scores on high quality metrics.
A novel post-processing module has been developed on this analysis, which is sort of a supercharged device for fixing pictures. It is designed to deal with extra complicated duties, like rebuilding the unique face and background, fixing up hair after mixing, and filling in any lacking elements. This module creates a very detailed picture, with 4 occasions extra element than what we used earlier than. In contrast to different instruments that target modifying pictures, ours prioritizes making the picture look nearly as good as potential while not having additional edits.
Demo
Carry this challenge to life
To run this demo we’ll first, open the pocket book HairFastGAN.ipynb
. This pocket book has all of the code we’d like try to experiment with the mannequin. To run the demo, we first must clone the repo and set up the mandatory libraries nevertheless.
- Clone the repo and set up Ninja
!wget https://github.com/ninja-build/ninja/releases/obtain/v1.8.2/ninja-linux.zip
!sudo unzip ninja-linux.zip -d /usr/native/bin/
!sudo update-alternatives --install /usr/bin/ninja ninja /usr/native/bin/ninja 1 --force ## clone repo
!git clone https://github.com/AIRI-Institute/HairFastGAN
%cd HairFastGAN
- Set up some essential packages and the pre-trained fashions
from concurrent.futures import ProcessPoolExecutor def install_packages(): !pip set up pillow==10.0.Zero face_alignment dill==0.2.7.1 addict fpie git+https://github.com/openai/CLIP.git -q def download_models(): !git clone https://huggingface.co/AIRI-Institute/HairFastGAN !cd HairFastGAN && git lfs pull && cd .. !mv HairFastGAN/pretrained_models pretrained_models !mv HairFastGAN/enter enter !rm -rf HairFastGAN with ProcessPoolExecutor() as executor: executor.submit(install_packages) executor.submit(download_models)
- Subsequent, we’ll arrange an argument parser, which is able to create an occasion of the
HairFast
class, and carry out hair swapping operation, utilizing default configuration or parameters.
import argparse
from pathlib import Path
from hair_swap import HairFast, get_parser model_args = get_parser()
hair_fast = HairFast(model_args.parse_args([]))
- Use the beneath script which incorporates the capabilities for downloading, changing, and displaying pictures, with help for caching and numerous enter codecs.
import requests
from io import BytesIO
from PIL import Picture
from functools import cache import matplotlib.pyplot as plt
import matplotlib.gridspec as gridspec
import torchvision.transforms as T
import torch
%matplotlib inline def to_tuple(func): def wrapper(arg): if isinstance(arg, record): arg = tuple(arg) return func(arg) return wrapper @to_tuple
@cache
def download_and_convert_to_pil(urls): pil_images = [] for url in urls: response = requests.get(url, allow_redirects=True, headers={"Person-Agent": "Mozilla/5.0"}) img = Picture.open(BytesIO(response.content material)) pil_images.append(img) print(f"Downloaded a picture of dimension {img.dimension}") return pil_images def display_images(pictures=None, **kwargs): is_titles = pictures is None pictures = pictures or kwargs grid = gridspec.GridSpec(1, len(pictures)) fig = plt.determine(figsize=(20, 10)) for i, merchandise in enumerate(pictures.objects() if is_titles else pictures): title, img = merchandise if is_titles else (None, merchandise) img = T.useful.to_pil_image(img) if isinstance(img, torch.Tensor) else img img = Picture.open(img) if isinstance(img, str | Path) else img ax = fig.add_subplot(1, len(pictures), i+1) ax.imshow(img) if title: ax.set_title(title, fontsize=20) ax.axis('off') plt.present()
- Strive the hair swap with the downloaded picture
input_dir = Path('/HairFastGAN/enter')
face_path = input_dir / '6.png'
shape_path = input_dir / '7.png'
color_path = input_dir / '8.png' final_image = hair_fast.swap(face_path, shape_path, color_path)
T.useful.to_pil_image(final_image).resize((512, 512)) # 1024 -> 512
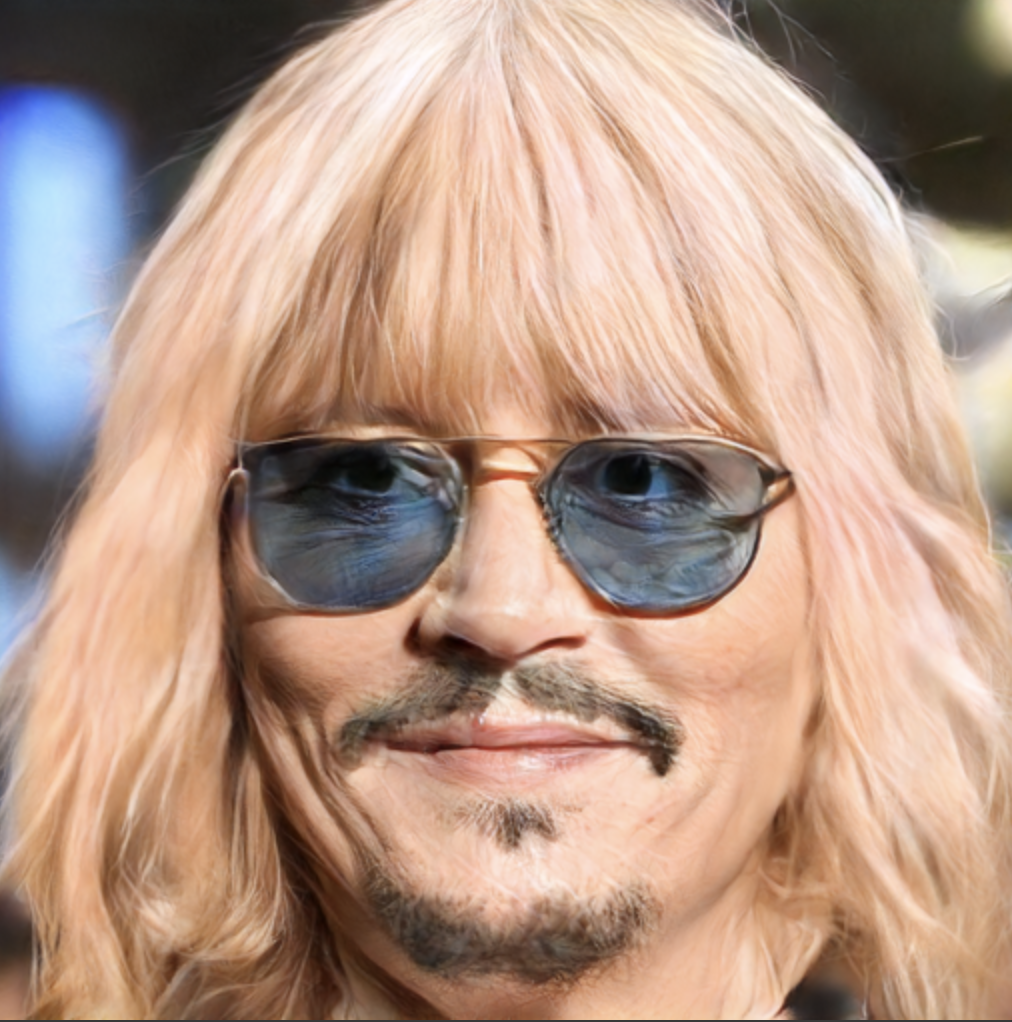
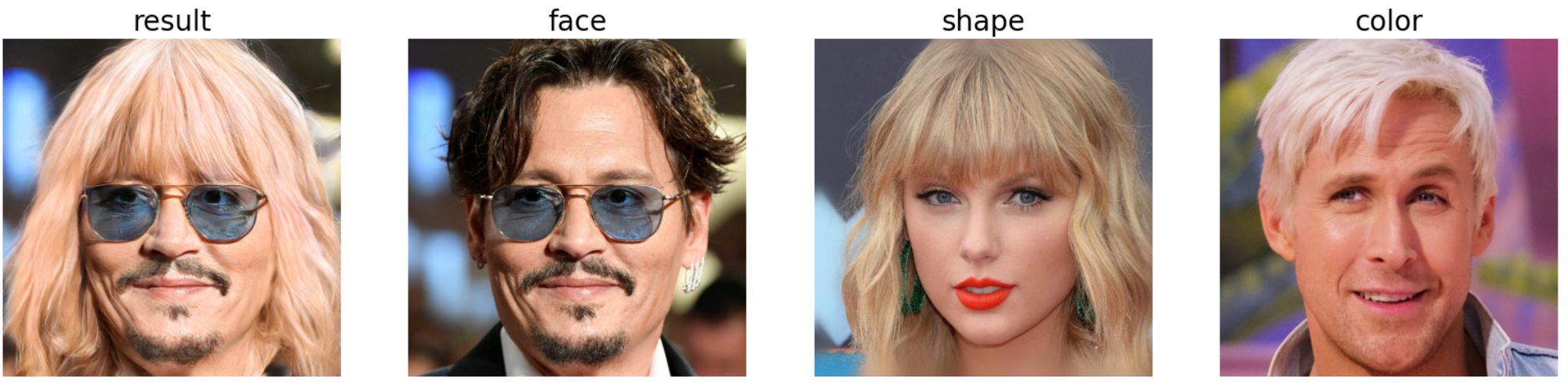
Ending Ideas
In our article, we launched the HairFast methodology for transferring hair, which stands out for its capacity to ship high-quality, high-resolution outcomes similar to optimization-based strategies whereas working at practically real-time speeds.
Nevertheless, like many different strategies, this methodology can also be constrained by the restricted methods to switch hairstyles. But, the structure lays the groundwork for addressing this limitation in future work.
Moreover, the way forward for digital hair styling utilizing AI holds immense promise for revolutionizing the way in which we work together with and discover hairstyles. With developments in AI applied sciences, much more real looking and customizable digital hair makeover instruments are anticipated. Therefore, this results in extremely customized digital styling experiences.
Furthermore, because the analysis on this subject continues to enhance, we are able to count on to see better integration of digital hair styling instruments throughout numerous platforms, from cellular apps to digital actuality environments. This widespread accessibility will empower customers to experiment with totally different appears to be like and developments from the consolation of their very own units.
General, the way forward for digital hair styling utilizing AI holds the potential to redefine magnificence requirements, empower people to precise themselves creatively and rework the way in which we understand and have interaction with hairstyling.
We loved experimenting with HairFastGAN’s novel strategy, and we actually hope you loved studying the article and making an attempt it with Paperspace.
Thank You!