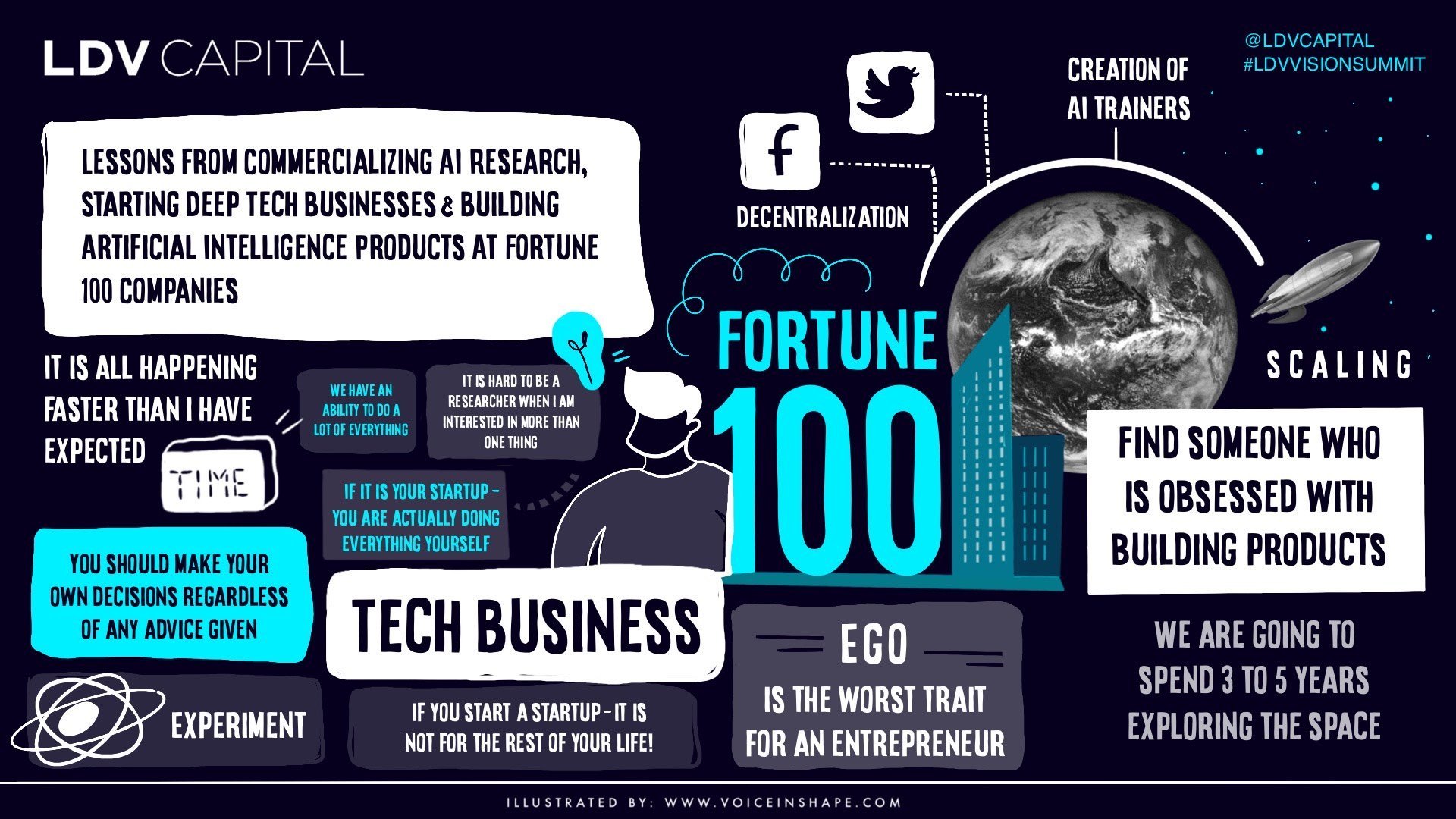
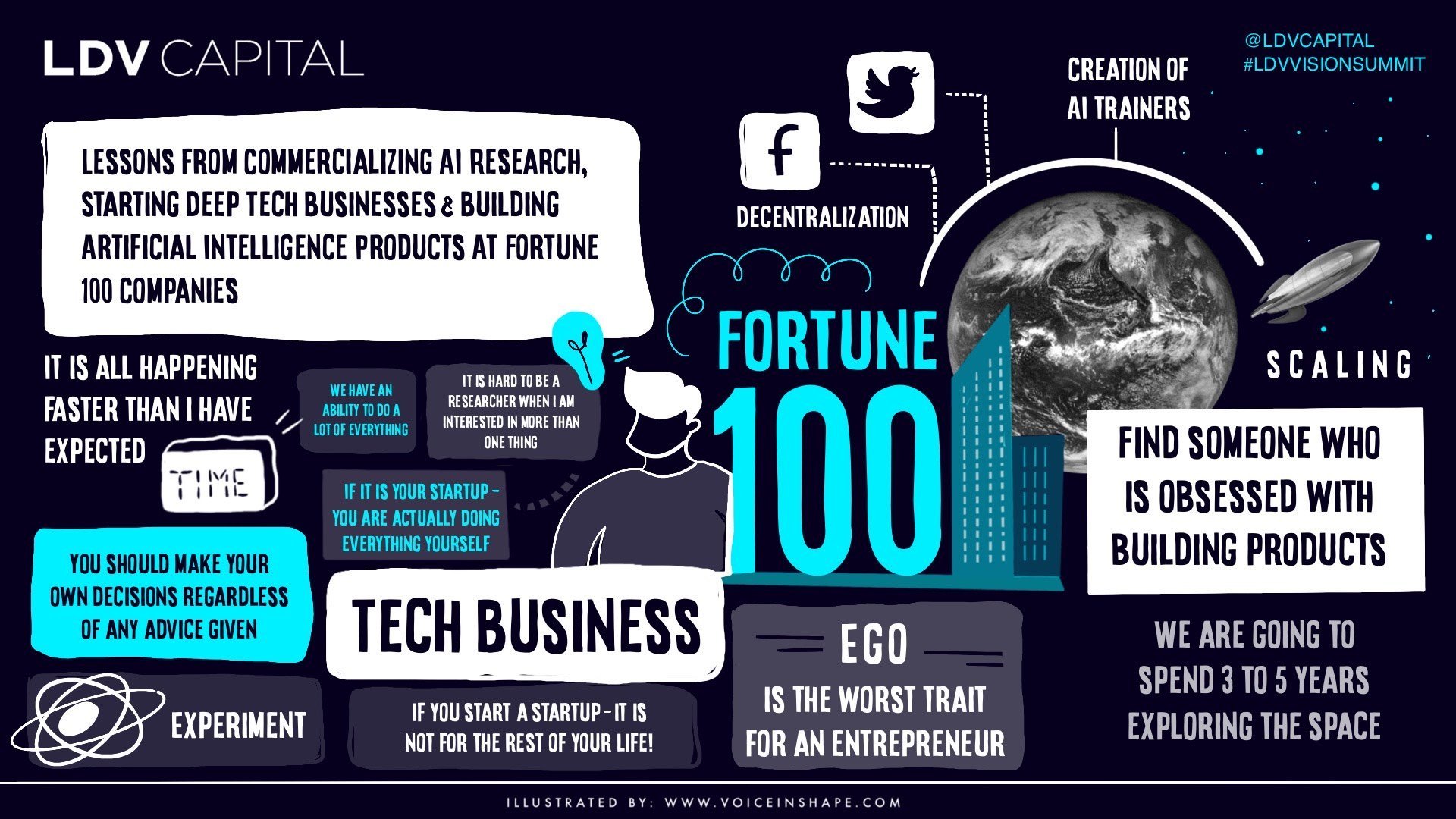
Interview with Clément Farabet, VP of Analysis at DeepMind
Clément Farabet obtained his Ph.D. from College Paris-Est whereas a visiting researcher at NYU. He was co-founder & CTO of Madbits.
The Madbits staff constructed visible intelligence know-how that mechanically understands, organizes and extracts related data from uncooked media. Understanding the content material of a picture, whether or not or not there are tags related to that picture, is a fancy problem. They developed their know-how based mostly on deep studying, an strategy to statistical machine studying that entails stacking easy projections to type highly effective hierarchical fashions of a sign. In 2014, Madbits was offered to Twitter, the place Clément then co-founded and led Twitter Cortex for nearly Three years.
Clément was VP of AI & AV Infrastructure at NVIDIA, the place he was constructing the foundations of NVIDIA’s AI, AV and Knowledge Science platforms, MagLev and RAPIDS.
Most just lately, Clément joined DeepMind to assist their extraordinary staff proceed to steer of their quest to construct synthetic normal intelligence (AGI).
If you happen to missed our ninth Annual LDV Imaginative and prescient Summit, that is your probability to observe the video or learn this shortened & frivolously edited transcript. You may also need to try our 5-question interview with Clement that was revealed main as much as the Summit together with 13 different interviews.
Evan: While you have been a Ph.D. scholar, what did you suppose you have been going to be doing in 10 years and has it occurred the way in which you thought?
Clément: Some 10-15 years in the past after I began working in direction of my Ph.D, I joined a small crew of individuals worldwide who have been obsessive about neural networks. We thought that neural networks can be the trail to AGI.
Again then, we did not use the time period AGI, however we used AI on the whole, and there have been 30-40 individuals worldwide who thought that scaling these neural nets can be a method to clear up general-purpose intelligence issues. Quick-forward 10 years from that point, I used to be satisfied that I might’ve contributed some key items to this AI puzzle. Did it pan out as deliberate? I am unsure. I contributed some essential issues to some massive corporations. We nonetheless have a protracted method to go, however yearly is getting increasingly thrilling on this exponential path to fixing this downside. It seems that neural nets have been certainly a key a part of the puzzle. I am completely satisfied about that!
Evan: How lengthy may one thing occur if we’re proper or if we’re improper? It sounds such as you suppose it might need occurred sooner, or do you suppose it is occurring slower or quicker than you anticipated once you have been a researcher?
Clément: It is all occurring quicker than I anticipated. In 2008-2010, plenty of the initiatives I used to work on have been to construct {hardware} accelerators for neural nets as a result of all of us knew that compute and the provision of nice coaching knowledge have been the important thing issues to scale these neural nets. It seems that these items are nonetheless true and related immediately.
Again then, constructing this kind of {custom} pc was key to creating any type of progress. Then NVIDIA took over this complete business they usually leaped us ahead by a lot! It’s nearly like a time machine. We might’ve waited for perhaps 20 years to have sufficient compute and sufficient knowledge to resolve these issues. All the things occurred method quicker!
Previously couple of years, we’re realizing that by scaling these fashions on pure textual content enter, we are able to study extraordinarily deep fashions of the world, which is stunning to many. It is nonetheless not fully solved. There are various different issues to determine, but it surely’s occurring quicker by at the very least 10 years, from my perspective.
Evan: That is nice as a result of I continuously really feel it takes longer than anticipated. I might reasonably it occur quicker as a result of then there’s extra time for iteration. This additionally means thrilling issues and that thrills me! How did you determine to begin Madbits as an alternative of becoming a member of an enormous firm?
Clément: Again then I could not determine whether or not I used to be going to be a researcher and dedicate my life to researching a few of these deep questions and determining these deep issues, or I used to be going to be an entrepreneur and begin corporations and determine a method to construct merchandise with that nice know-how and convey that to the market. I’ve a hyperactive nature, and so I wish to be engaged on many issues directly. It is onerous to be a researcher when you’ve got this mindset as a result of it requires extra concentrate on doing one factor at a time and doing it actually in-depth.
Throughout my Ph.D., I began two different software program corporations earlier than Madbits. Madbits was the time when all the pieces began to make sense to me. I knew that we wanted to proceed investing in deep tech, however some massive corporations have been going to do this higher than a startup.
It was additionally the right timing for these applied sciences, particularly visible neural networks as a result of a lot of the massive corporations didn’t have any know-how to have a look at visible content material. Pictures & movies have been largely invisible to them. Firms like Twitter, Apple, and even Fb again then have been coping with metadata. It was the right timing to construct a startup that will construct a visible search engine. I bought enthusiastic about that.
We spent two years constructing the core know-how, and we had one of many first merchandise – visible search. We listed a lot of the social media streams, which again then have been public.
Evan: The place was that workplace?
Clément: On the Decrease East Facet.
Evan: There have been 5 of you in a small workplace for 2.
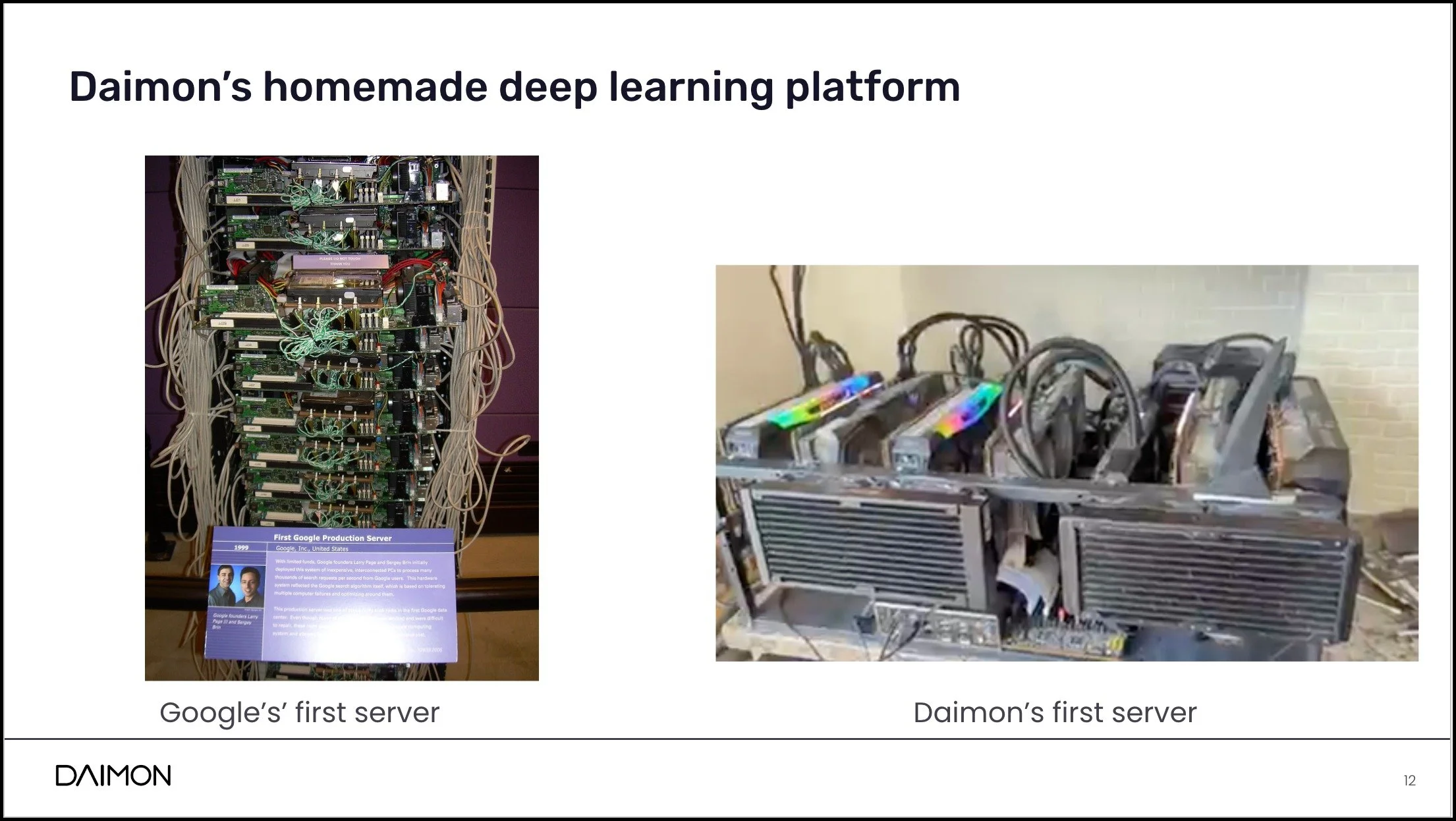
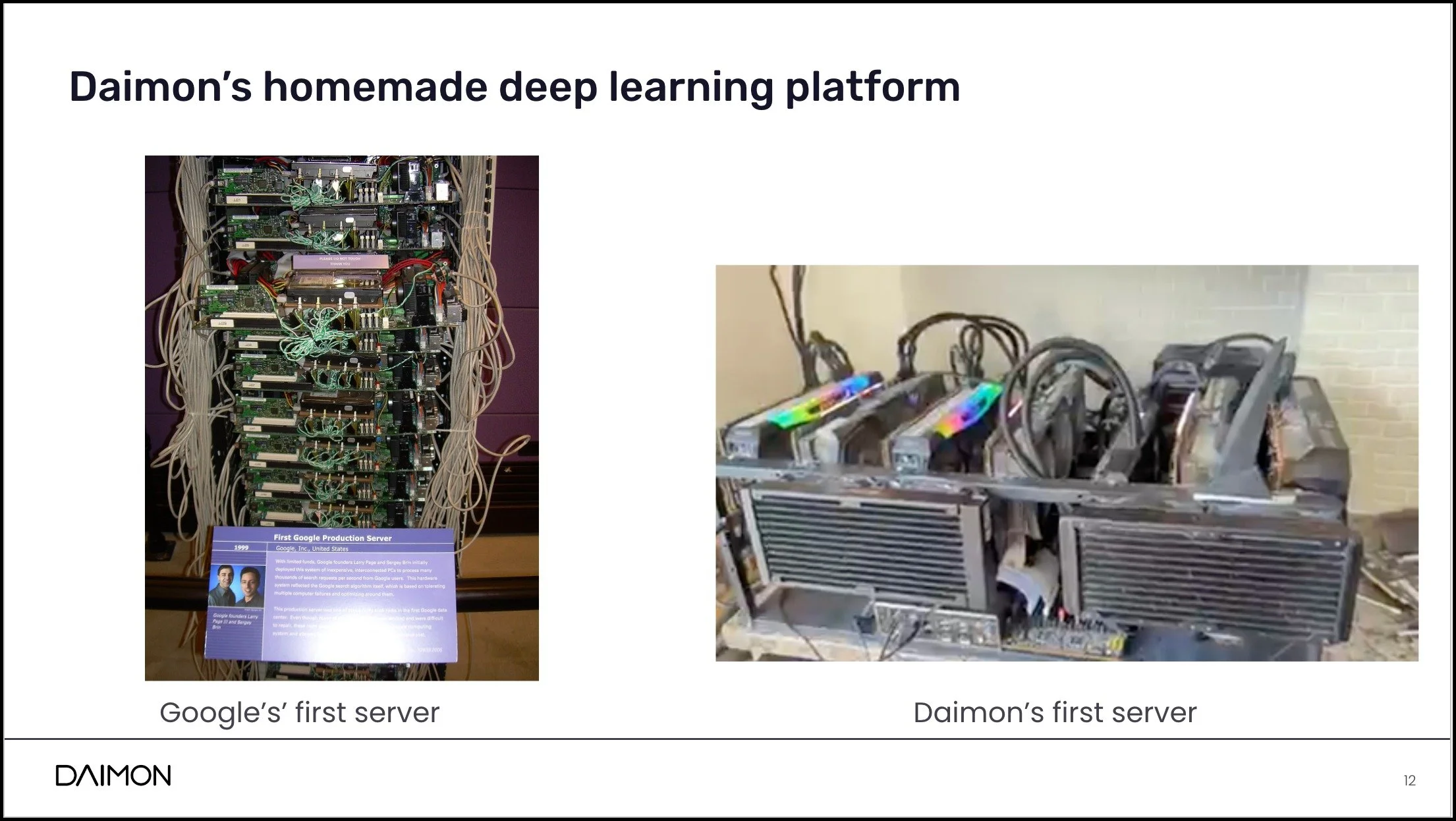
Clément: That is proper. I simply watched the earlier discuss, “The Subsequent Era of Massive Language Mannequin Chatbot with Human-Like Empathy”, which was nice, and this kind of custom-built GPU rigs… we had the identical factor! I assembled your entire GPU rig we had. All the things was NVIDIA-based. It was within the again workplace of that small residence constructing, and we took down the electrical energy for your entire constructing at the very least 4-5 occasions within the span of 6 months.
Evan: Once I was an entrepreneur, it was pre-cloud computing. We have been managing thousands and thousands of photos of which we have been coaching or working the servers within the workplace earlier than we went to screw them into the co-location facility. There have been solely two of us. That is a part of each startup.
Clément: Precisely. To your earlier query, that is what I like as effectively. As an entrepreneur, you begin an organization, you will be doing all the pieces. You are actually going to be cleansing the ground of that residence your self.
Evan: I used to be the top sweeper for the hallway! What was Madbits and why did you promote it to Twitter?
Clément: Madbits was a visible search engine firm, and we by no means bought it to the market. It remained a closed beta. This factor was so spectacular! You might search by a particularly massive dataset of visible content material, photos, movies, and your private library. We might index your Apple images. We might index the net and social media. We showcased our working prototype to some executives, 5-6 most important corporations in Silicon Valley. We heard, “We need to purchase you, we have to have that as a part of our backend,” and that is what occurred.
Evan: While you joined Twitter, you constructed this staff referred to as Cortex Core, and I discovered an article titled, “Twitter’s new AI acknowledges porn, so you do not have to”. Inform me how that relates and is that what you have been hoping they have been going to publish? Was that what you have been doing?
Clément: That title is absolute click-bait and we discovered that when it bought revealed. I keep in mind having an interview, speaking about these applied sciences and being enthusiastic about what we have been constructing. Then this factor got here out and I used to be like, “Come on…”.
Evan: Clearly, what you have been constructing is rather more highly effective than that, but it surely was a part of what you have been serving to to handle that content material moderation, proper?
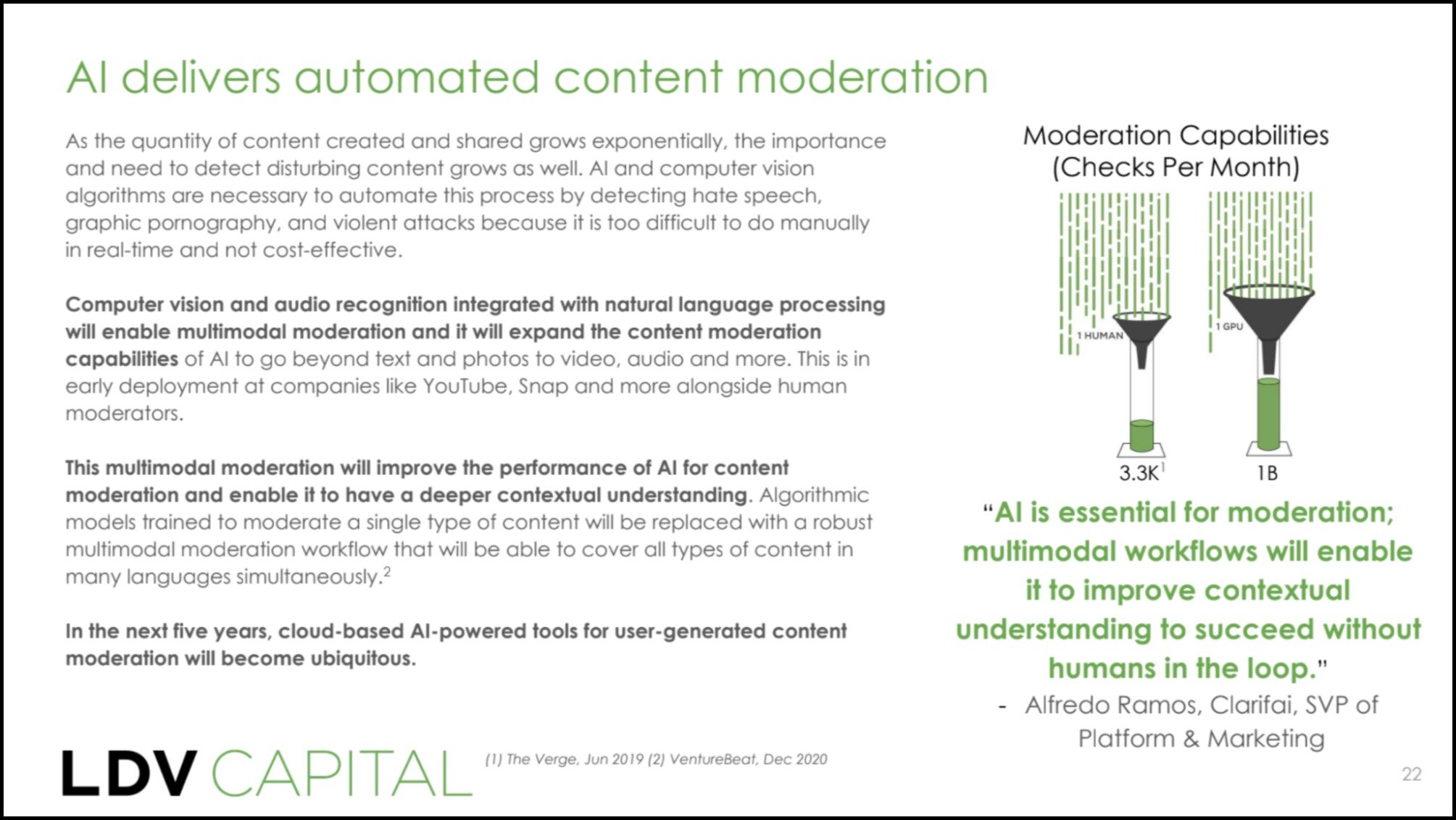
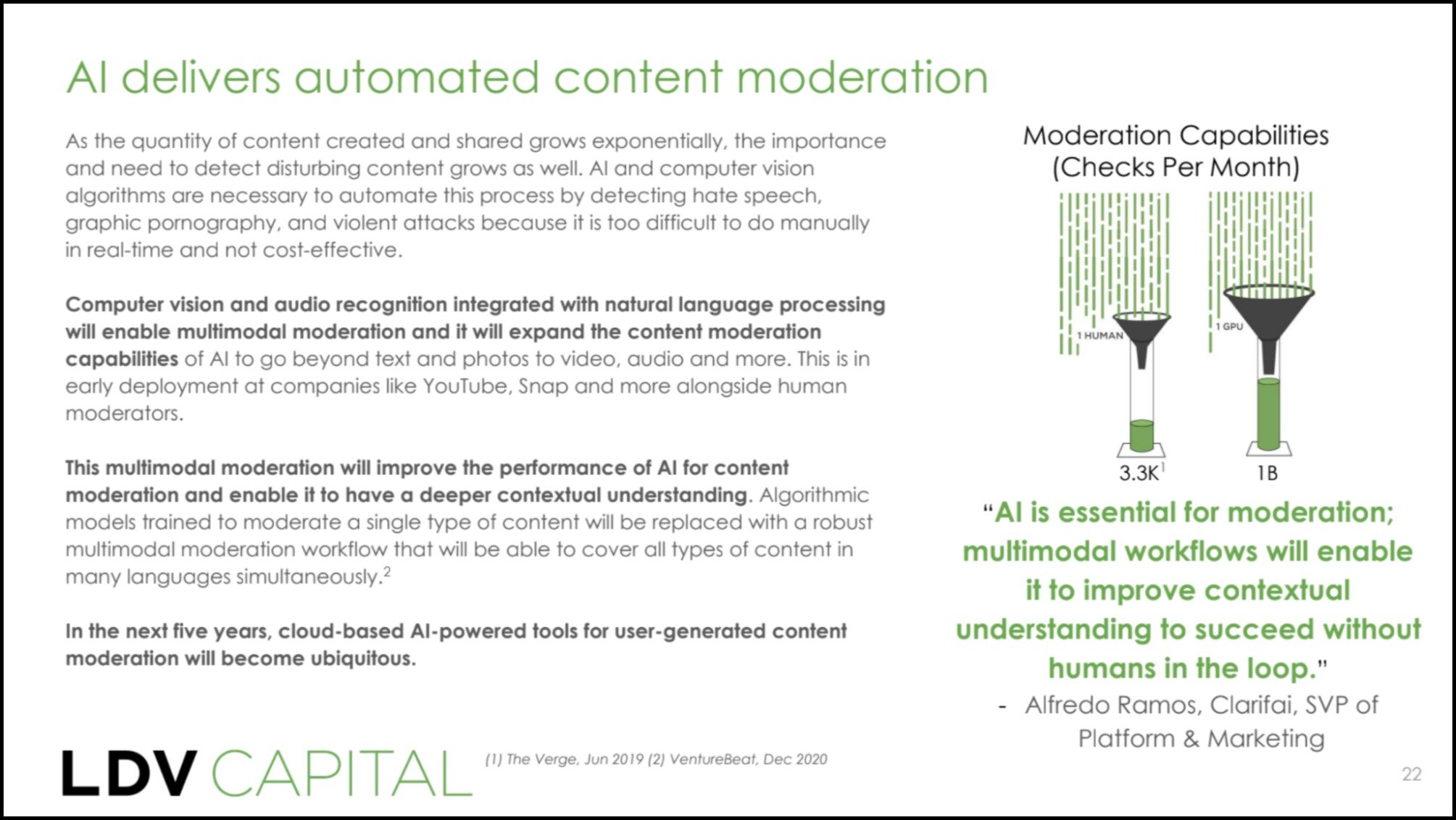
In our 2021 LDV Insights report, “Content material & the Metaverse are Powered by Visible Tech”, we examined the highest visible tech tendencies which can be reshaping digital creation and recognized the distinctive enterprise alternatives to help creation over the subsequent 5 years.
Clément: Click on-bait title apart, the article was spot on. Massive-scale social media platforms have unbelievable moderation challenges, largely as a result of plenty of them determined to centralize content material moderation. Platforms like Twitter or Fb are constructed round this concept that there can be some centralized entity that will determine what could be showcased in public areas versus what must be down-ranked.
Primarily based on that, it’s essential to depend on these forms of algorithms to look into content material and assist filter out issues that will make no sense for the better viewers. These issues have been gnarly and never so nice to have a look at, but it surely felt good to resolve them as a result of they helped make these platforms safer areas. I am glad we did it. The press-bait half was not so nice although.
Evan: So that you’re at Twitter, which is an enormous firm, after which how’d you make that call of becoming a member of NVIDIA, one other huge firm?
Clément: I like what we constructed at Twitter however this firm essentially lacked technical management and imaginative and prescient when it comes to what to do subsequent. Round that point, I met Jensen Huang, the co-founder of NVIDIA, and we began having lengthy chats round what’s the way forward for AI. It was 6-7 years in the past, and we have been describing what’s occurring immediately, which is mind-blowing! Jensen at all times had this super skill to see the longer term, at the very least on some form of 5-10 yr horizon and place all his investments in order that they’d develop into the platform for that. He succeeded at that. It was an excellent technique and alignment with what the business wanted. I bought enthusiastic about becoming a member of him and constructing one thing that was extra elementary than my work at Twitter. Cortex was the platform for AI at Twitter however was like a facet product. At NVIDIA, I had the chance to go construct that for your entire business and apply that to deeper know-how issues like self-driving automobiles, as an example.
Evan: So far as problem and alternative, that’s large! Was it what you anticipated?
Clément: Partly, sure. NVIDIA is on the absolute heart of this complete know-how revolution we’re going by. You’ll be able to have a look at each startup that is doing generative AI or attempting to use AI to any of what we’re doing immediately, more than likely they’re consuming NVIDIA know-how at one degree of the stack.
I anticipated to begin constructing a consumer-facing AI platform for NVIDIA. Very quickly, it turned rather more essential for us to construct an inner platform to help new initiatives like self-driving automobiles, genomics and so forth. I went again to constructing background infrastructure, and it was nice. We constructed one thing super and that’s meant to proceed scaling within the subsequent years to come back. I’m pleased with that.
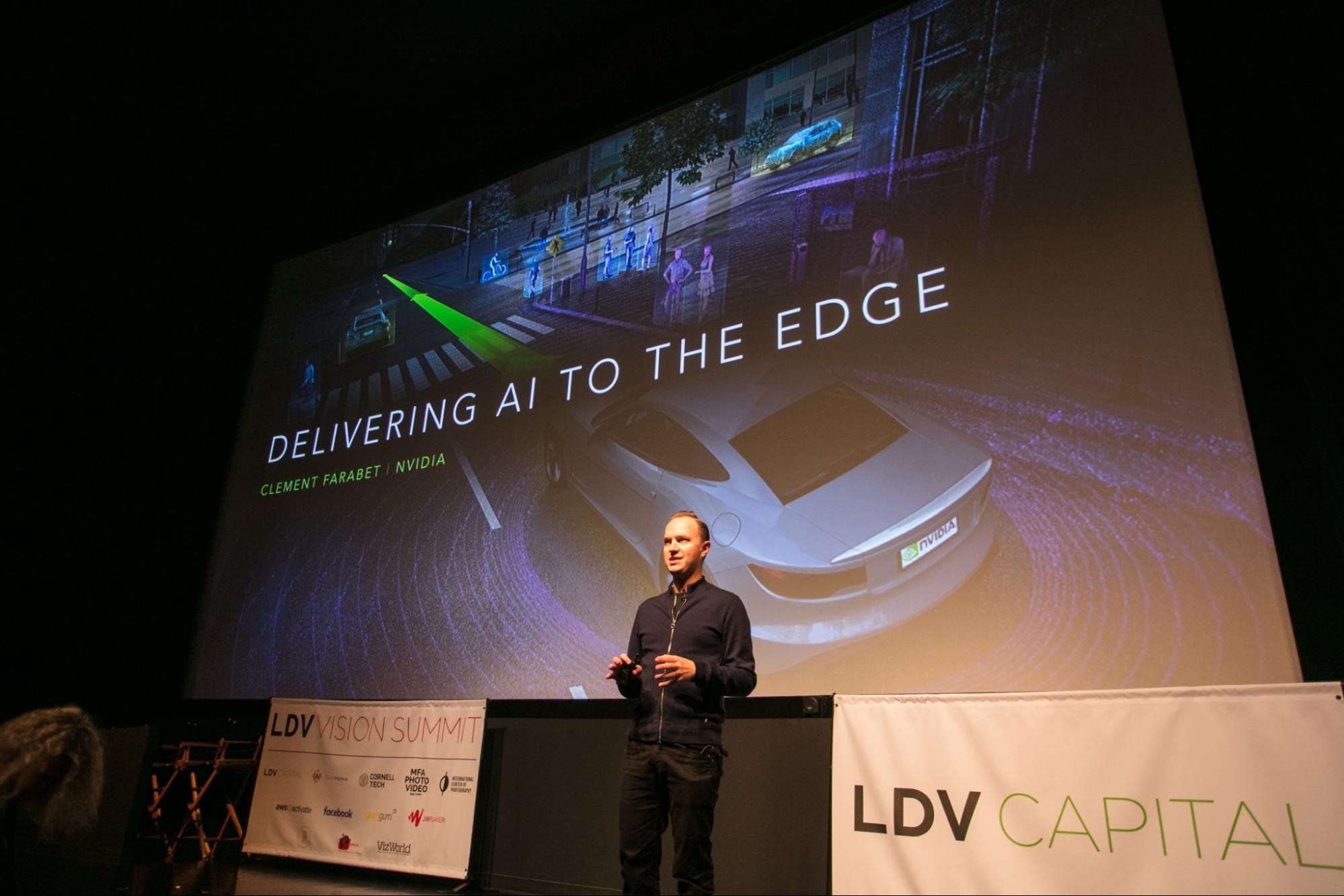
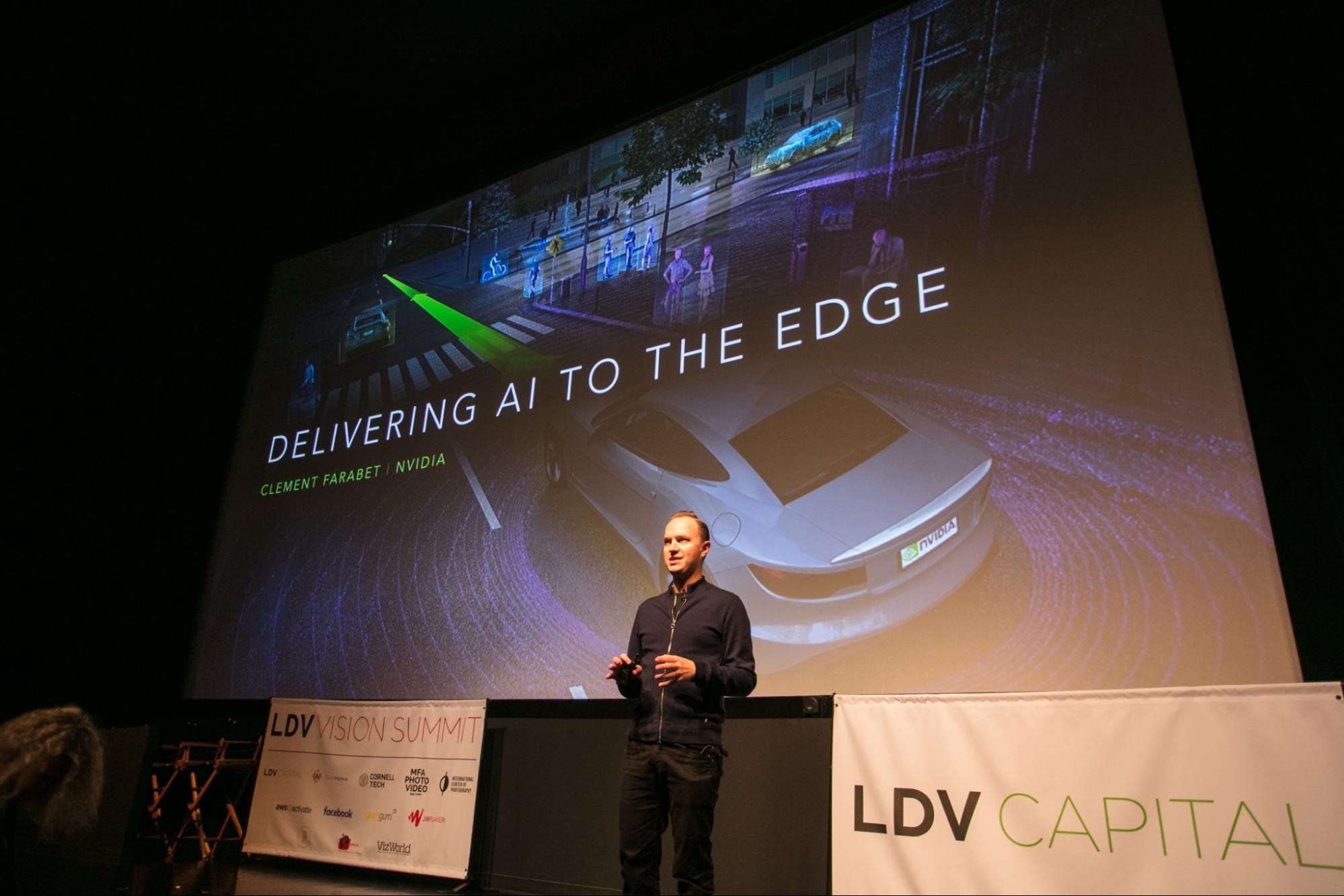
I wished to return to the product and be extra related to the purposes we’re constructing and the way AGI is coming collectively as a complete. That is what led me to maneuver on and discover new alternatives. NVIDIA is a implausible firm and I want good luck to anyone who’s attempting to compete with them.
Evan: While you introduced leaving NVIDIA, there was an in-between interval, and I assumed you may begin a brand new firm, however you determined to affix DeepMind. Let’s discuss this transition. Every time a buddy, an excellent particular person goes out and in of massive corporations or thinks about beginning a brand new firm, it is at all times attention-grabbing to know or to learn the way every of them makes selections. It looks like you like each the startup world and the massive corporations. However Twitter, NVIDIA and DeepMind, it is three huge corporations in a row, proper?
Clément: Once I transferred over from Twitter to NVIDIA, I had no second ideas. That was such a no brainer.
At NVIDIA, I additionally operated as an entrepreneur in some sense as a result of we created this challenge referred to as Rapids which is NVIDIA’s open-source knowledge sense platform. We ran it as a startup.
I left NVIDIA and took a 3+ month break. I began an organization in that timeframe, and I assumed that that was what I used to be going to do. The corporate was targeted on attempting to automate the entire technique of constructing knowledge units for the coaching of AI brokers. It is one in all our largest elementary issues proper now that should be solved at scale.
The creation of datasets is probably the most impactful factor to coach great-quality AI.
Evan: Plenty of startups are attempting to do this or say they’re doing that, Clément. I assume you are saying that it is nonetheless not being executed effectively.
Clément: It is a particularly onerous downside. Arguably even for the good labs and corporations like Open AI a lot of that course of is handbook immediately. Plenty of people are wanting on the failure modes and deciding what to do subsequent, which works to an extent. We’re creating higher and higher AI like that however we have got to have the ability to do higher.
I had a chat with Demis Hassabis, DeepMind’s founder and CEO, a buddy of mine. I labored for him 10+ years in the past after I was nonetheless doing my Ph.D. earlier than Madbits. I began describing the place I used to be at, and what I used to be attempting to resolve, and he satisfied me shortly that DeepMind has all these nice know-how parts and on the cusp of constructing one thing even better. I’ve such an amazing position as a part of that!
If AI was not occurring at this scale proper now, I might in all probability be again to being a startup entrepreneur and determining what to construct subsequent.
I am torn as a result of I am so in love with the know-how and the basic rules of scaling AI know-how, that I really feel like doing it with all these companions at that scale is the place I should be.
Evan: It sounds prefer it’s a transparent choice, but it surely’s attention-grabbing that you simply dipped your toes again within the water. Perhaps you had to do this to know whether or not or not that was the suitable subsequent step. Typically we’ve got to! Earlier than I began LDV Capital, I began one other challenge and invalidated it shortly. I in all probability did not know I needed to scratch that itch to make it possible for it wasn’t the suitable factor to do.
Clément: That is proper. I might have constructed one thing by myself once more. However then there’s this large development coming now of the scaled basis fashions, and I am glad that there is plenty of the ecosystem now that is attempting to breed that both in open supply or with completely different methods or producing extra compact variations of that. I need to bounce into this core downside.
Are massive language fashions (LLMs) enough or are we going to wish extra and the way will we get to the subsequent step?
Evan: What are you going to be engaged on at DeepMind? What are the challenges you are excited to work on?
Clément: We now have plenty of issues that we’re planning on doing, however I do not need to focus on a few of these issues too early.


LLMs are a key a part of the puzzle. We name them LLMs now, they’re simply scaled neural networks with this nice transformer-based structure that treats enter streams as tokens. These fashions have demonstrated that being educated on an enormous quantity of textual content knowledge, they’re in a position to construct an inner mannequin of the world that is satisfying and that can be utilized to question them. That is spectacular!
We now have numerous unsolved issues. We have to construct one thing that is extra cohesive, full, and able to getting near AGI. We’ll spend the subsequent 3-5-10 years (I do not understand how lengthy it may take us) exploring the remainder of the house.
Evan: How does the researcher, the Ph.D., make the suitable choice to both begin a enterprise or go to an enormous firm?
Clément: It is such a private choice! You get enter from individuals, it is good to get recommendation, and it is good to have mentors. However on the finish of the day, you make your individual selections, and it is essential that you simply make them based mostly on rules that apply to you.
Evan: It looks like many deep technical researchers really feel prefer it’s nearly binary. You’ve got one alternative or one other. I see it as an iterative course of. We now have plenty of completely different chapters in our profession, however I do not know, as a result of I am not a researcher or an engineer, I simply collaborate with them rather a lot. What do you consider that?
Clément: It is true. When you’re youthful, 20+, you are getting out of college, you are feeling like the choices you will make are going to outline your total profession, and it isn’t true in any respect. We stay in a world that is so dynamic and you’ll experiment a lot! You’ll be able to go in 3-5 yr chunks. It is essential to do plenty of all the pieces!
Once I began my firm, I had no expertise in huge tech. Now that I do, I really feel like I might be a a lot stronger younger entrepreneur as a result of I perceive a number of the methods these massive organizations work and the true state of know-how.
While you get out of grad faculty, you are naive but it surely’s additionally your energy as a result of you do not know what you do not know. That pushes you to do issues which can be somewhat loopy.
For researchers particularly: do you need to work on elementary analysis for a great chunk of your profession? If the reply is sure, you need to persist with it early on as a result of it is onerous to get again in. If you happen to begin transferring into enterprise and creating corporations, you are by no means going to get again into elementary analysis. That is an essential choice.
If you happen to’re dancing round between utilized analysis in an industrial context, or constructing merchandise, you’ve got a lot freedom and you’ll commute. Try to be experimental.
Evan: What’s the most important mistake you made as an entrepreneur?
Clément: I made 3-Four main errors. Considered one of them was freely giving an excessive amount of fairness early on.
Evan: To founders or to buyers?
Clément: Traders. I used to be naive, I did not totally perceive the subsequent steps. 10-15 years in the past, there was a lot much less road data round the entire fundraising course of from Seed to Collection A. Now it is so codified that people have rather a lot to learn. Again then, it was tougher. We guessed rather a lot and I did some issues that put us in a state of affairs the place it was higher for me to exit early than attempt to construct it out. If I hadn’t made that mistake, I might in all probability have tried tougher.
Evan: What do you suppose is the very best and worst trait of an entrepreneur?
Clément: The worst trait is ego. The very best trait is grift.
Evan: What visible applied sciences are you most excited to lastly seem or exist in 20 years?
Clément: I spent the previous 6 years constructing the core know-how for self-driving automobiles. We made super progress there! On this subject, I’ll agree with what you mentioned earlier, this area moved method slower than anticipated.
Evan: It is true however is not it due to human nature versus vehicles? The know-how is there, however the automation shouldn’t be.
Clément: In some sense, driving a car has such a security problem that it is nearly an AGI downside, the place you’ll need to have an agenda that may totally perceive, have an amazing world mannequin of all the pieces that surrounds it and might purpose about it in methods which can be much like the way in which we do it.
At this time, we’re nonetheless brute forcing plenty of these layers. We have decomposed the issue and we’re counting on the dimensions in neural nets to resolve plenty of that downside, which works nice, but it surely’s not taking us to the end line. Firms like Cruise and Waymo have solved this downside with this loopy costly sensor platform. With the lidar, cameras, and radars constructing SD maps forward of time, they’ll clear up the issue in finite environments, like one neighborhood in Phoenix and one neighborhood in San Francisco, the price to scale it to each different neighborhood on the planet is insane. It is in all probability going to take us greater than a decade to get there.
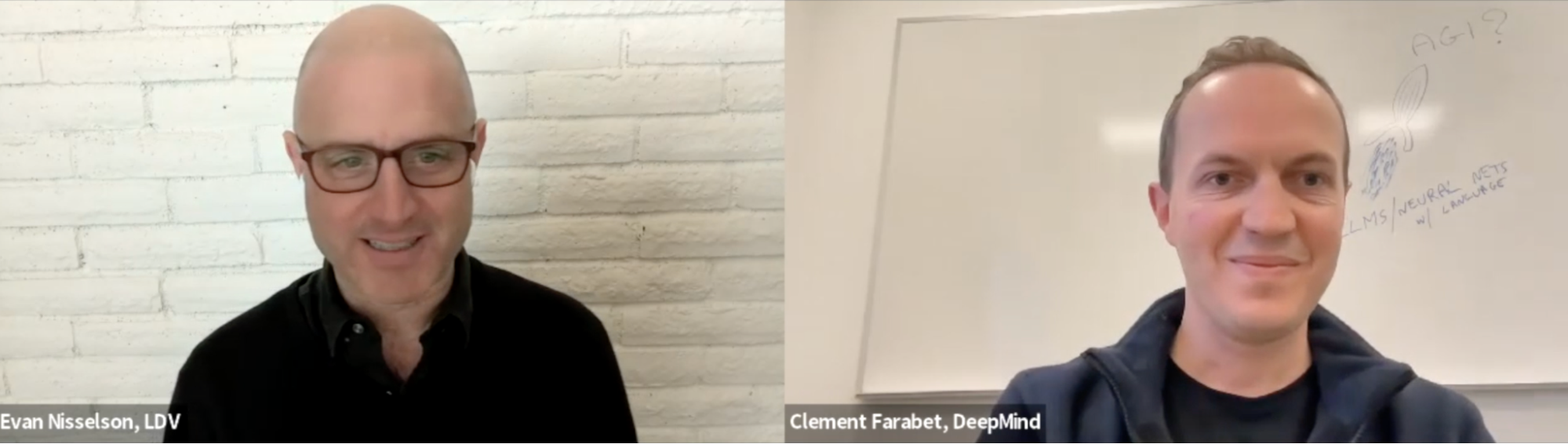
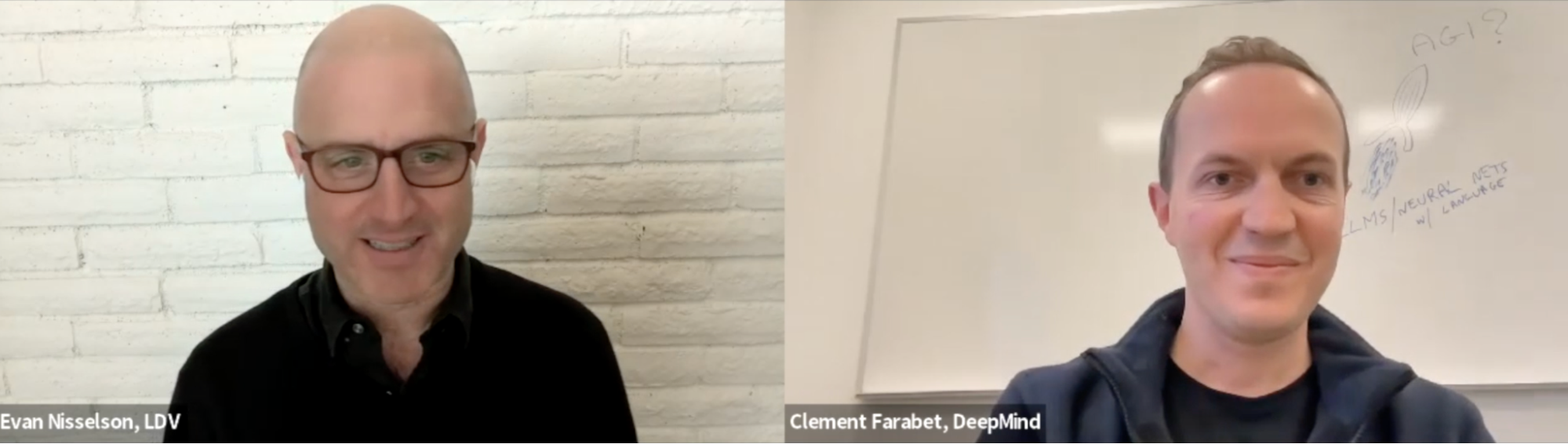
Within the meantime, we’ll have cracked some extra elementary items of that AGI puzzle in order that we are able to get to true world fashions. One thing that is pretty much as good as LLMs, however for the visible world. Then we’ll begin leaning on that to resolve the issue.
LLMs bought quicker, however take into account that they do have the identical sort of boundary circumstances issues the place you could possibly not deploy an LLM immediately in a safety-type surroundings. There’s an excessive amount of unknown! They are going to do issues which can be bullshit generally and you do not know it.
Evan: What’s one final piece of recommendation for researchers that need to construct companies? Apart from going to LDV.
Clément: Speak to Evan for positive. He is an amazing man!
The principle recommendation might be the one I gave to myself again then: discover somebody who loves constructing merchandise, has an incredible product sensibility, and is obsessive about that factor that’s going to please prospects.
Neural nets helped plenty of corporations like Open AI and DeepMind, however once you construct an organization, you need to construct a product that persons are in love with. Discovering a associate like that’s the key factor.