CogVLM is a Giant Multimodal Mannequin (LMM) to which you’ll be able to ask questions on photographs and textual content. For instance, think about a situation the place you might be aiming to determine baggage on the tarmac in an airport, a possible security hazard. You might take common images of a given part of tarmac and ask CogVLM “is there baggage on the tarmac?”
On this information, we are going to talk about what CogVLM is and stroll by means of just a few use instances for CogVLM in business. We’ll talk about utilizing CogVLM to implement airport security precautions, monitoring for faulty containers, and figuring out characters in a picture.
With out additional ado, let’s get began!
What’s CogVLM?
CogVLM is a multimodal mannequin that solutions questions on textual content and pictures. CogVLM, not like many multimodal fashions, is open supply and will be run by yourself infrastructure. In our testing, CogVLM carried out properly at a spread of imaginative and prescient duties in our testing, from visible query answering to doc OCR.
With Roboflow Inference, you may deploy CogVLM with minimal guide setup. Inference is a pc imaginative and prescient inference server with which you’ll be able to deploy a spread of state-of-the-art mannequin architectures, from YOLOv8 to CLIP to CogVLM.
Inference lets you run CogVLM with quantization. Quantization compresses the mannequin, permitting you to run the mannequin with much less reminiscence necessities (albeit with a slight accuracy trade-off). Utilizing 4-bit quantization, you may run CogVLM on an NVIDIA T4 GPU. In our testing, requests with this configuration take round 10 seconds to course of.
To learn to deploy CogVLM by yourself infrastructure, check with our information on easy methods to deploy CogVLM.
CogVLM Use Circumstances in Business
You’ll be able to deploy a server or a number of servers inside your group to make use of CogVLM.
CogVLM is right for visible query answering the place you could have a picture and also you need to ask a query in regards to the picture.
CogVLM is especially helpful when the query you might be asking is complicated, comparable to “is there a employee near a conveyor belt?” or “is there baggage on the tarmac?” Within the first case, you may leverage the mannequin’s potential to grasp how objects relate. Within the latter case, you may leverage the mannequin’s potential to generalize to completely different variants of an object (baggage), a problem for object detection fashions as a result of customized logic is required.
Given the slower processing occasions for multimodal fashions like CogVLM, we suggest utilizing the mannequin to be used instances that can not be simply solved by fine-tuned object detection and classification fashions. Positive-tuned fashions will be run by yourself {hardware} at (near) actual time efficiency, relying on the computing assets accessible.
Let’s discuss by means of just a few use instances to which CogVLM might be utilized.
Implementing Security Precautions
When airport employees transfer baggage onto a airplane, it’s important no baggage is left on the runway. This can be a security hazard. Historically, you would wish to coach a baggage detection mannequin. This could take time given the massive variation in types and colours of baggage in addition to various digital camera angles or distances of objects within the body.
You’ll be able to ask CogVLM “is there baggage on the tarmac” to determine if there may be baggage on a tarmac. Such an answer might be deployed when you construct an correct, fine-tuned mannequin that works to your use case.
We requested CogVLM “Is there baggage on the airport tarmac?”, with the next picture:
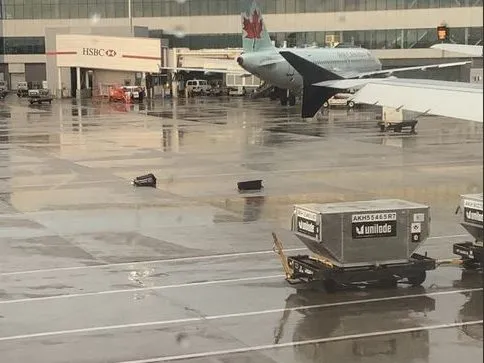
To which the mannequin responded:
Sure, there are just a few items of baggage scattered on the airport tarmac.
CogVLM efficiently recognized there was baggage on the tarmac, a security hazard.
Monitoring for Product Defects
Think about a situation the place it is advisable to guarantee the standard of merchandise in a producing facility. Parcels which were broken – dented, for instance – must be flagged. The next incidence charge of dented parcels could point out that there’s an upstream drawback in packaging or one other a part of the distribution pipeline that must be addressed.
You might take images of parcels and ask CogVLM “is that this package deal dented?” or “does this package deal have a defect?” It will permit you to flag any potential points. You too can use the output from CogVLM to assemble metadata that you need to use to raised report the difficulty the mannequin has recognized. For instance, if the mannequin recognized a dent in a field, you may report that there was a dent given the response returned by the mannequin.
For instance, we requested CogVLM “Is the package deal dented?” with the next picture as context:
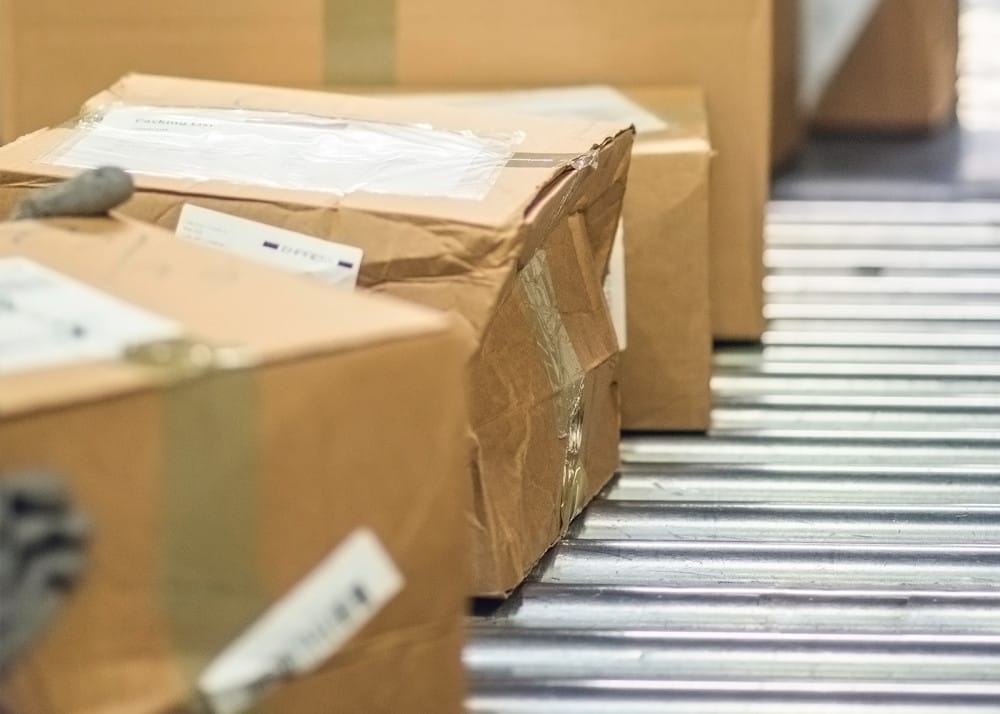
The mannequin responded:
Sure, the package deal seems to have a dent or a crease on its aspect.
CogVLM efficiently recognized the parcel as broken.
With that mentioned, coaching your personal fine-tuned object detection mannequin could also be superb over time. This mannequin can work in actual time, not like CogVLM. You might use CogVLM to gather knowledge on faulty packages that you would be able to then label to be used in coaching a fine-tuned object detection mannequin.
Optical Character Recognition
You too can use CogVLM for Optical Character Recognition (OCR). Whereas a conventional OCR mannequin could possibly help, the efficiency of such fashions varies relying in your use case. When you’ve got struggled to retrieve correct outcomes from conventional OCR fashions, we suggest experimenting with CogVLM.
For instance, think about a situation the place it is advisable to learn the serial quantity on a automobile tire. You need to use CogVLM to retrieve characters on the tire. For one of the best accuracy, we suggest working the mannequin with out quantization.
We requested CogVLM to learn the serial quantity on the next tire, with the immediate “Learn the serial quantity.”:
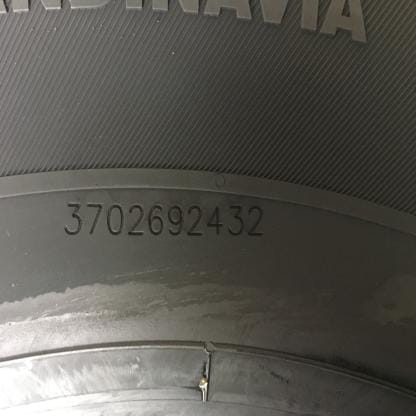
The mannequin returned the next response:
The serial quantity on the tire is 3702692432.
CogVLM efficiently learn the serial quantity.
Conclusion
CogVLM is a multimodal mannequin that may reply questions on photographs and textual content.
On this information, we walked by means of three functions of CogVLM in business: figuring out security hazards, monitoring for product defects, and OCR.
CogVLM will be deployed in your infrastructure, not like different multimodal fashions comparable to OpenAI’s GPT-Four with Imaginative and prescient and Google’s Gemini. You’ll be able to run CogVLM with quantization to make use of the mannequin with much less RAM. There’s a tradeoff in accuracy, however you must consider if the tradeoff is important sufficient to make use of a bigger mannequin in testing to your use case
In case you are able to deploy CogVLM by yourself infrastructure, check with our CogVLM deployment information. This information walks by means of the whole lot it is advisable to deploy CogVLM.